category
🚀 People ask me what it takes to build a scalable Large Language Model (LLM) app in 2023. When we talk about scalable we mean 100s of users and millisecond latency. Let me share some of our learning experience with you.
1. Architecture:
The emerging field of LlmOps is not only about accessing GPT-4 and GPT-3.5 (or an LLM), it is a complete echo-system where you need to have a knowledge center (where your LLM can get up to date knowledge), data pipelines (where you digest text/non-textual data), Caching ( when you scale you want to save costs) and playground. One of the best reads so far on architecture is from a16z that I totally recommend before diving into the next sections [1].
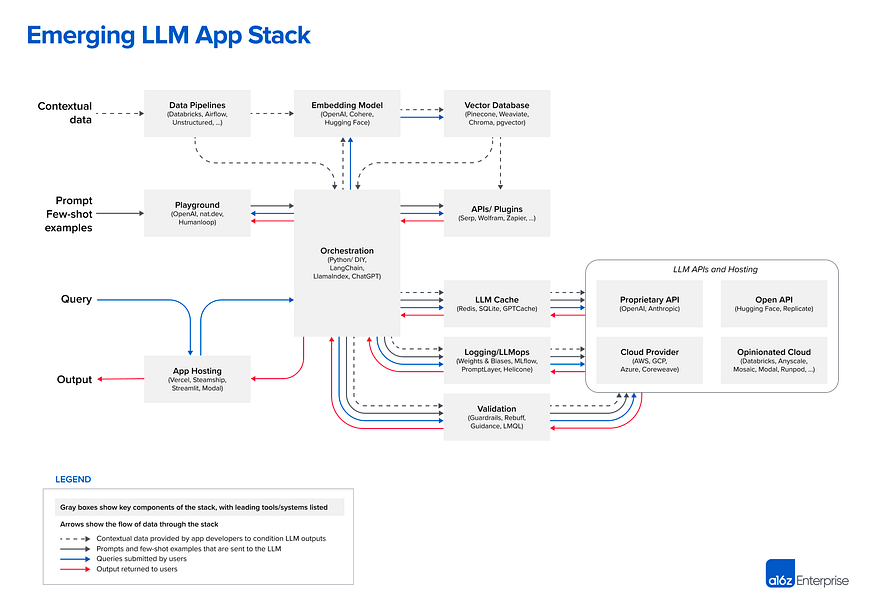
2. Backend ( Python Vs No-Python):

Save your team time and effort and use python (if you want to build on top of the state of the art work in NLP). Thanks to FastAPI [2] (props to Sebastián Ramírez Montaño), you can now build asynchronous apps with very small latency.
“[…] I’m using FastAPI a ton these days. […] I’m actually planning to use it for all of my team’s ML services at Microsoft. Some of them are getting integrated into the core Windows product and some Office products.” Kabir Khan — Microsoft [3].
Some of the very interesting features of FastAPI:
- Fully asynchronous ( it can scale to many users)
- Easy integration with relational databases such as SQL through SqlModel [4]
- Support for WebSockets (important for chatbots) and Server Sent Events (SSE — important for streaming responses) — More on this on the next articles
3. LangChain:

You will need to use Langchain one way or another depending on your application. However, our experience is that you cant just build scalable apps using Langchain out of the box. Many components in Langchain as of today are not asynchronous by nature and while you see many tutorials telling you, you can create an app in 3 lines of code, trust me your app wont be scalable. The most important NON-async components (as of today) in LangChain are vector-stores (retrievers) and Tools (SerpAPI, etc).
What do we mean by Tools?
According to Langchain docs: Tools are interfaces that an agent can use to interact with the world.
It is often relatively straightforward to subclass tools in Langchain and add an asynchronous module to them.
How about vector-stores (retrievers)?
Vector-stores are essential for many modern LLM apps. We leverage vector-stores to provide knowledge access to our LLM (more on this in the next section).
4. Knowledge-Base (VectorStore)
You see Linkedin Heros using LangChain and Chroma to build a Doc Q&A in a few lines of code. The reality could not be further from the truth. Vectorstores such as Chroma while easy to use have multiple issues. First, their Langchain integration is sync (not scalable). Second, these vectorstore’s latencies are not low enough to scale to many users.
Our experience is that you are better off using a vendor provided VectorStore, if you don’t have sensitive data (e.g. pinecone) or deploy your own.
In the later case, Redis (if you aim to have users in range of 100s) and Qdrant (more than 1000s- written in Rust), due to the fact that they are low latency, provide great search functionalities, scalable and very easy to integrate.
I recommend leveraging async implementation of most of the vector-stores from ChatGPT-Retrieval plugin.
5. Caching:

Caching and saving costs are crucial for your application as you scale. There are great libraries out there such as GPTCache that offer semantic caching. Semantic caching is useful when you want to cache semantically similar prompts.
Though, I dont recommend using GPTCache if you are using Redis in your stack. You might be better off building your own using Redis Asyncio and its integrated vectorstore.
6. Validation and Robustness of responses:
Where there is no guidance, a model fails, but in an abundance of instructions there is safety.
- GPT 11:14
Microsoft Guidance is a great tool for constrained generation. What does constrained generation mean you ask? It means you can force a language model to generate responses in a more predictable way. This is extremely helpful if you use use LLMs as an Agent to use other tools.
7. Document Digestion:

Document Digestion is no easy task, since there are many file formats that you often need to support in your document for digesting knowledge. As of today, I recommend leveraging unstructured.io that automates all of these under a single API.
In the next series of my articles, I try to delve into each component of the LlmOps architecture in more details.
Stay tuned!
- 登录 发表评论